When it comes to implementing AI workloads, one of the most crucial decisions you'll face is choosing between on-premises infrastructure and the cloud. This decision can have far-reaching implications for your organization's bottom line, performance, security, and compliance. In this blog, we'll explore the economics, considerations, and factors that can help you make an informed decision.
The Cloud vs. On-Premises Dilemma
The cloud offers unparalleled flexibility, scalability, and agility. With pay-as-you-go pricing models, you can spin up resources on-demand, making it ideal for projects with fluctuating computational needs. On the other hand, on-premises infrastructure provides greater control over your hardware and data, giving you the ability to customize your environment to meet specific requirements. While the cloud's flexibility is appealing, it's essential to evaluate your organization's needs carefully. Consider factors such as the nature of your AI workloads, long-term cost implications, and data sensitivity before making a decision.
Economics of AI Workloads
Running AI workloads in the cloud can seem cost-effective initially, but costs can escalate as your data and computational needs grow. The pay-as-you-go model means that you pay for every resource you consume, and data transfer costs can add up, especially when dealing with large datasets.
For certain workloads, on-premises infrastructure can be more cost-effective over time, particularly if your AI projects require substantial computational resources continuously. To make an informed decision, perform a total cost of ownership (TCO) analysis, factoring in hardware costs, operational expenses, and long-term scalability.
Data Gravity: The Game-Changer
Data gravity is a concept that has been gaining traction in the world of AI and cloud computing. It refers to the idea that data, like a massive celestial body, exerts a gravitational pull, making it more challenging to move. This gravitational force can significantly impact your decision between on-premises and cloud infrastructure.
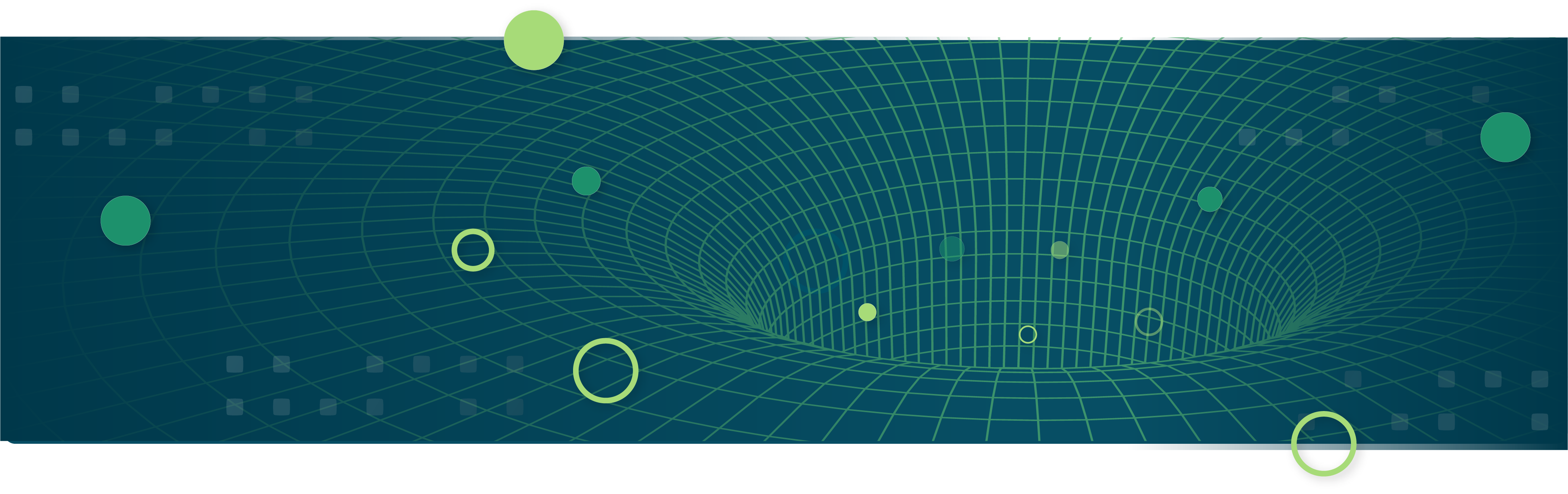
Imagine your organization has accumulated vast amounts of data over the years. This data has inertia, making it challenging and expensive to transfer from on-premises to the cloud or vice versa. Data location and transfer costs can be substantial barriers when dealing with data gravity. In such cases, it may make more sense to keep where the data resides.
Latency and Performance
Latency is another crucial factor in AI workloads. For applications that require real-time or near-real-time processing, hosting AI workloads in the same environment where the data resides will provide superior performance. This is because data doesn't need to travel over the internet to reach your computing resources, resulting in lower latency. Certain industries, such as autonomous vehicles or healthcare, demand low-latency AI solutions. In these cases, hosting AI workloads near the data becomes a compelling choice to ensure the quickest response times.
Security and Compliance
Security and compliance considerations can't be overlooked, especially in industries with stringent regulations like finance or healthcare. On-premises infrastructure provides greater control over data and allows you to implement customized security measures to meet specific compliance requirements. If your organization deals with sensitive data or must adhere to strict regulatory frameworks, the control offered by on-premises infrastructure may outweigh the benefits of the cloud.
Hybrid Solutions
In some cases, the best solution may not be an either/or decision. Hybrid solutions, combining the benefits of both on-premises and cloud infrastructure, can offer a middle ground. This approach allows you to keep sensitive data on-premises while leveraging the cloud's scalability for less sensitive workloads.
Ultimately, navigating the cloud vs. on-premises dilemma for AI workloads requires a deep understanding of your data, budget, and performance requirements. While the cloud offers flexibility and scalability, on-premises infrastructure provides control and cost-effectiveness, especially in the face of data gravity. By carefully evaluating these factors, you can make the correct business decision that propels your AI projects to success.
Before making a decision, thoroughly assess these factors and consider consulting with experts in AI infrastructure to ensure your choice aligns with your long-term business goals.
Categories
- Cloud Migration and Adoption
- Enterprise IT and Infrastructure
- Artificial Intelligence and Machine Learning
- Data Management and Analytics
- DevOps and Automation
- Cybersecurity and Compliance
- Application Modernization and Optimization
- Featured
- Managed Services & Cloud Cost Optimization
- News
- Workplace Modernization
- Tech We Like
- AWS
- Social Good News
- Cost Optimization
- Hybrid Cloud Strategy
- NVIDIA
- Application Development
- GPU
- Nonprofit