Insights
Technology Blog
- Data Management and Analytics
- Cloud Adoption
- Enterprise Infrastructure
- AI/ML
- DevOps
- Application Modernization
- Kubernetes
- Featured
- Dell EMC
- Google Cloud Platform (GCP)
- Multi-Cloud Operations
- Workplace Modernization
- Enterprise IT and Infrastructure
- Cloud
- Microsoft Azure
- Security & Governance
- News
- Tech We Like
- Amazon Web Services (AWS)
- IoT and Edge
- Security
- Artificial Intelligence (AI)
- SUSE Rancher
- Azure Security
- redapt
- migration
- CloudHealth
- Intel
- Snowflake
- Social Good
- AMD
- Azure Kubernetes Service (AKS)
- Generative AI
- Hybrid Cloud
- NVIDIA
- TimeXtender
- containers
- optimization
- CoreStack
- Customer Lifecycle
- Data Storage
- Elastic Kubernetes Service
- GPU
- Hardware
- Machine Learning (ML)
- Managed Services
- Storage
- VMware
- xIoT
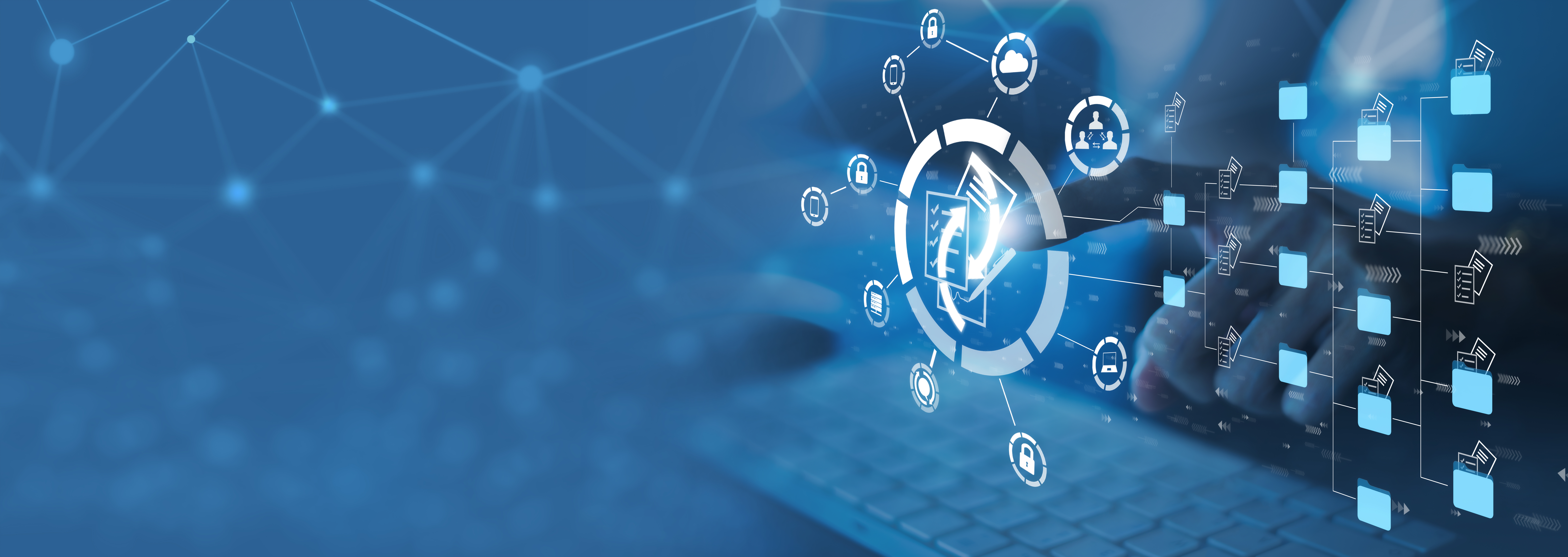
What is the Role of Artificial Intelligence in Business in 2024?
Artificial Intelligence (AI) is evolving faster than any other technology.
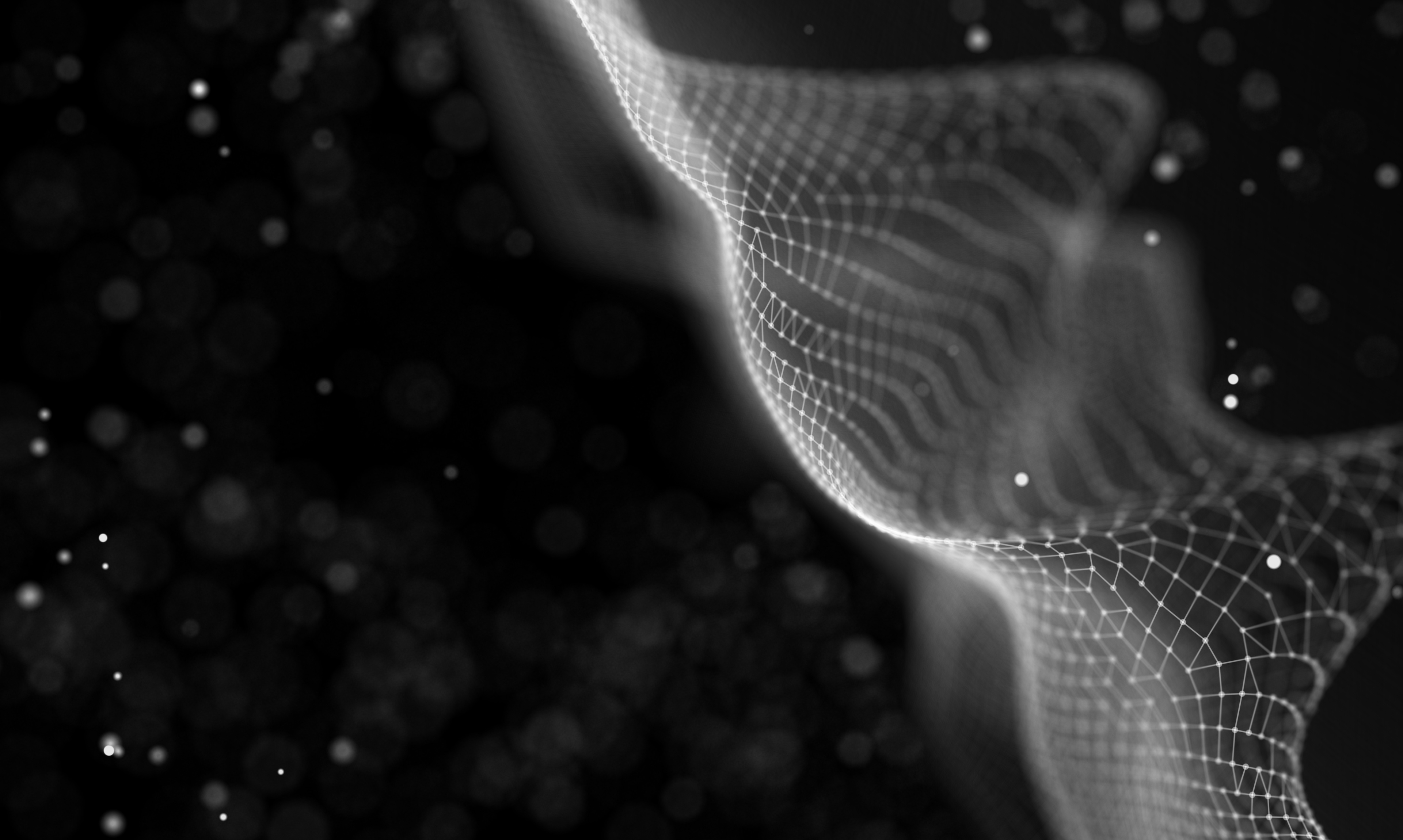
How to Use AI Workflow Automation to Amplify Results
Injecting AI into daily tasks can boost outcomes - but only if thoughtfully added to help your team rather than simply replacing them. 85 percent of leaders want to automate processes to increase...
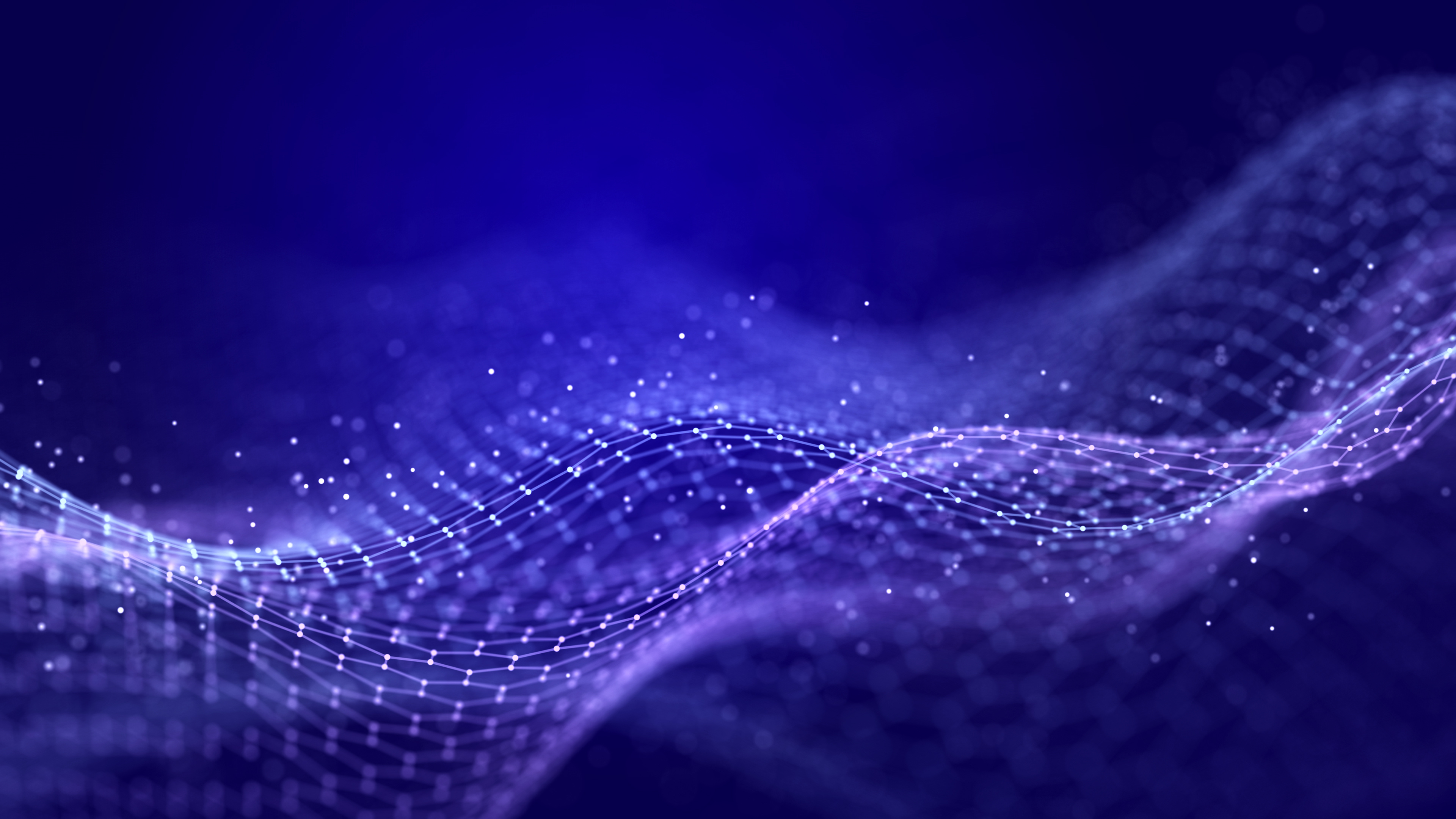
From Pilot to Production: A 5-Step Preparation Guide Before Putting AI to Work
46 percent of AI projects never make it to production.
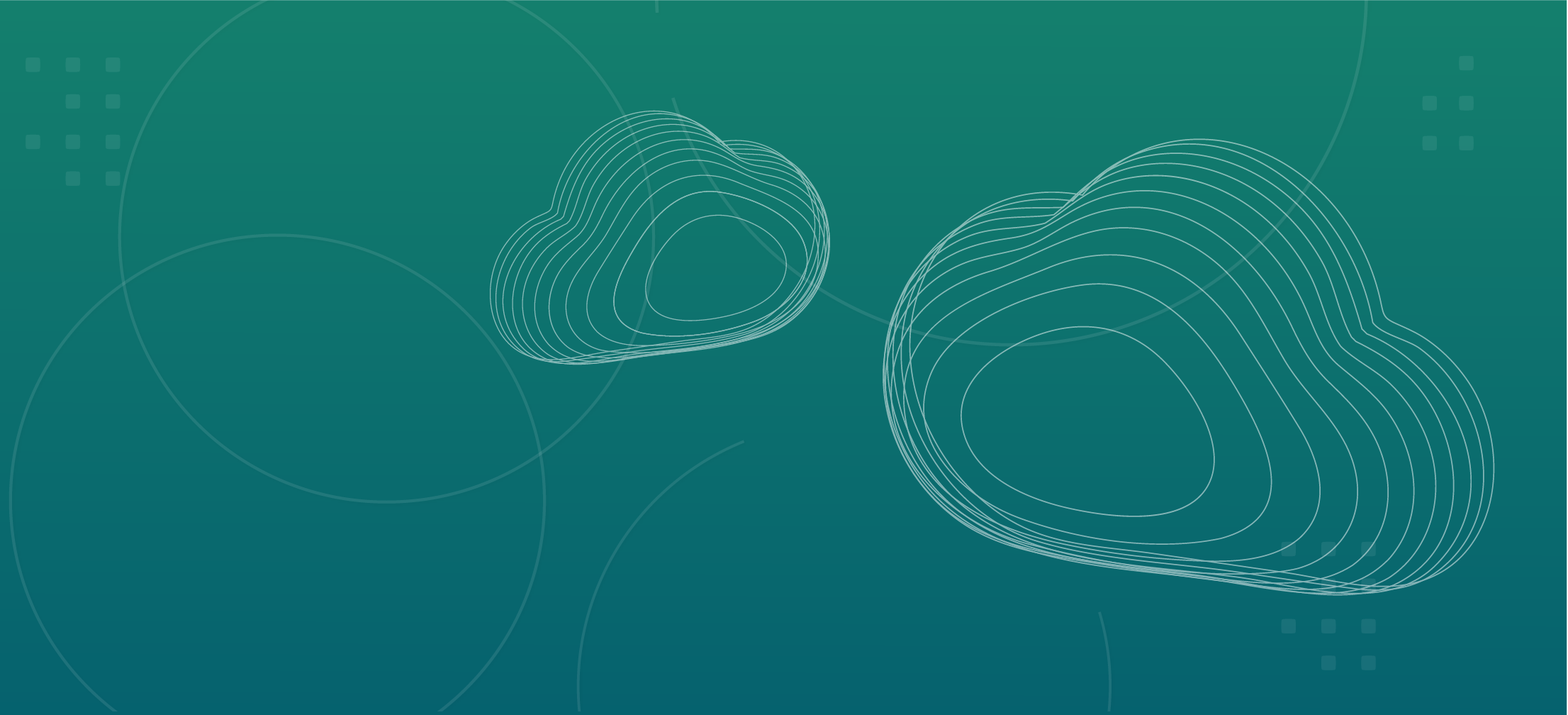
Announcing Redapt's Strategic Acquisition of Prime Foray: A New Era of Growth and Opportunities
Redapt is thrilled to announce its acquisition of Prime Foray, symbolizing our ambition and commitment to being a full-service technology solutions partner.
WOODINVILLE & BELLEVUE, WA — April 11, 2024
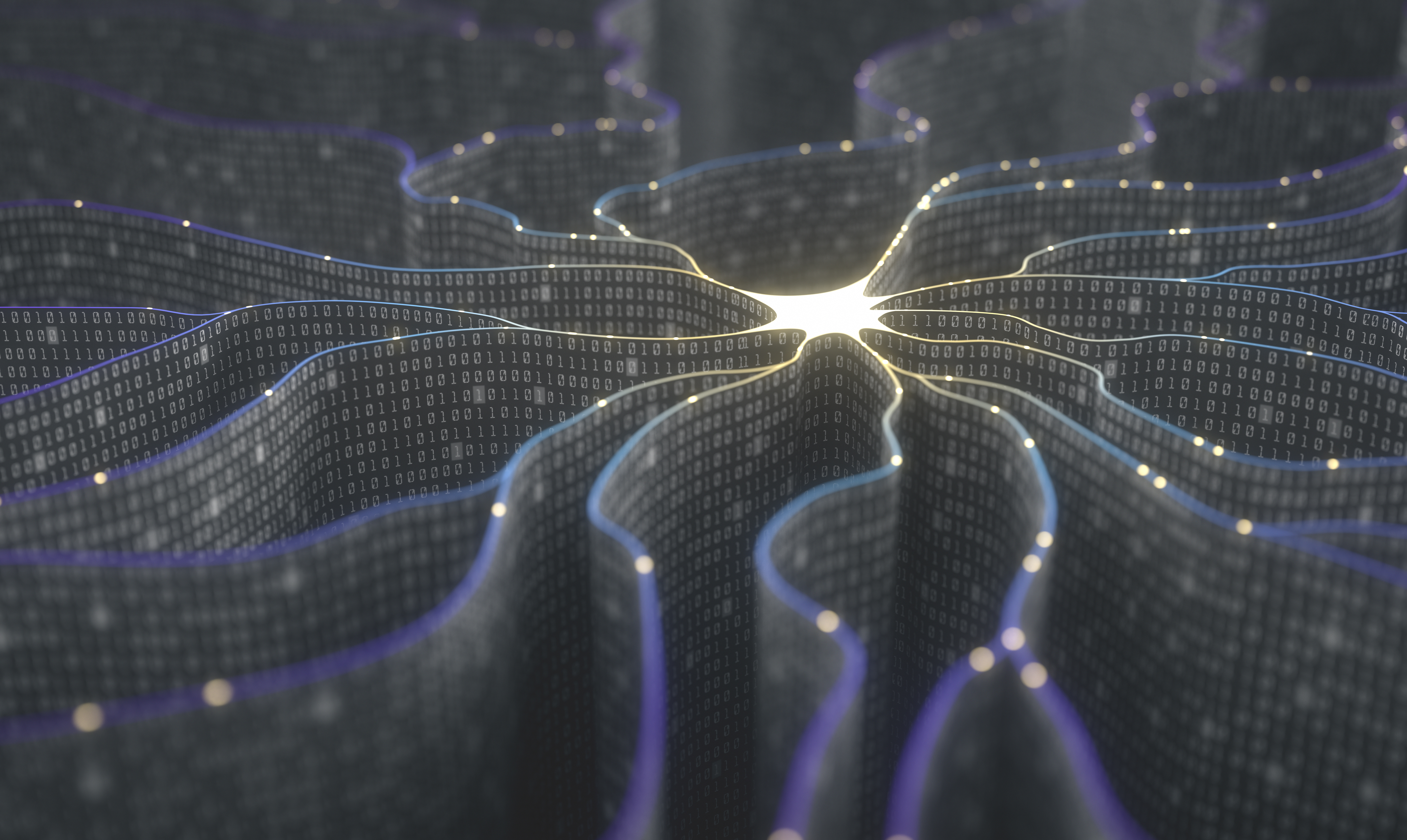
5 Benefits of AI in Business in 2024 and Beyond
Artificial intelligence adoption has exploded by 300% percent in the last five years. While basic automation has many advantages, advanced AI promises much more - from coordinating complex global...
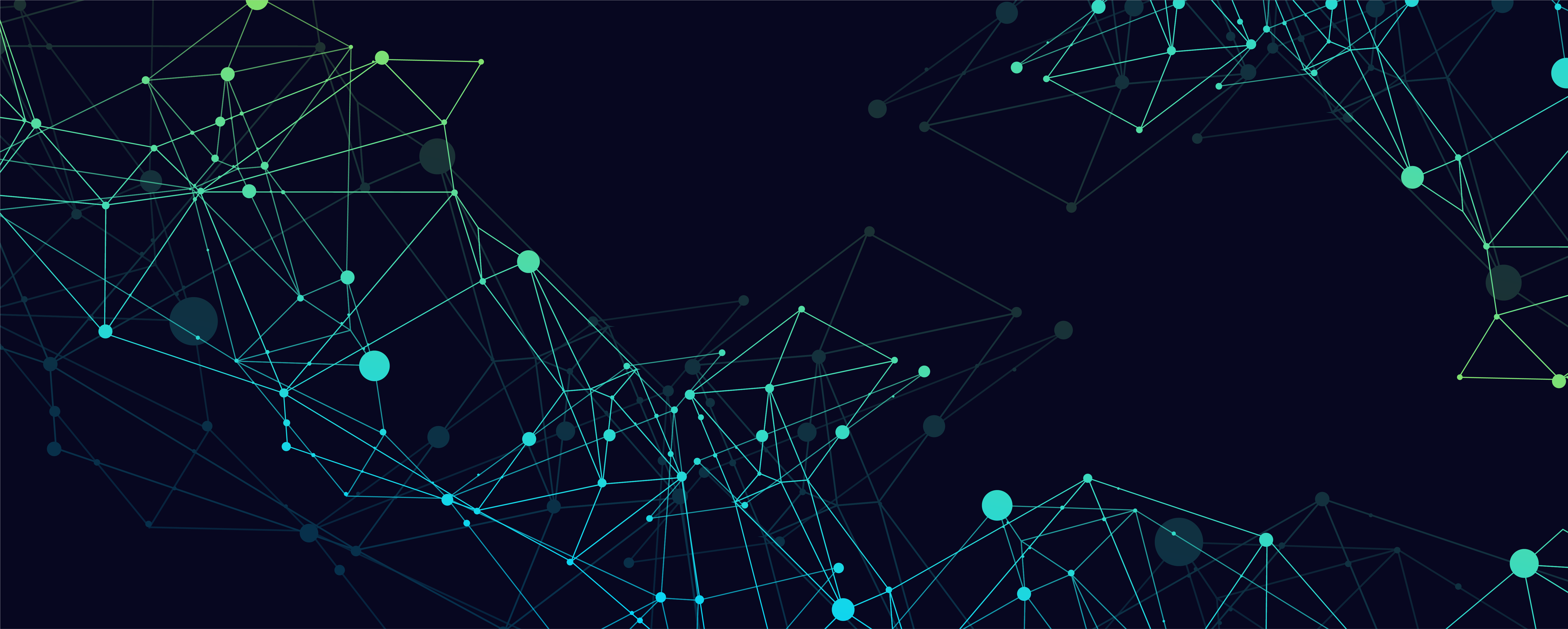
Get to Know the Well-Architected Framework Assessment
The flexibility, scalability, and cost-effectiveness offered by the cloud have made it indispensable for companies across industries. That’s the good news.
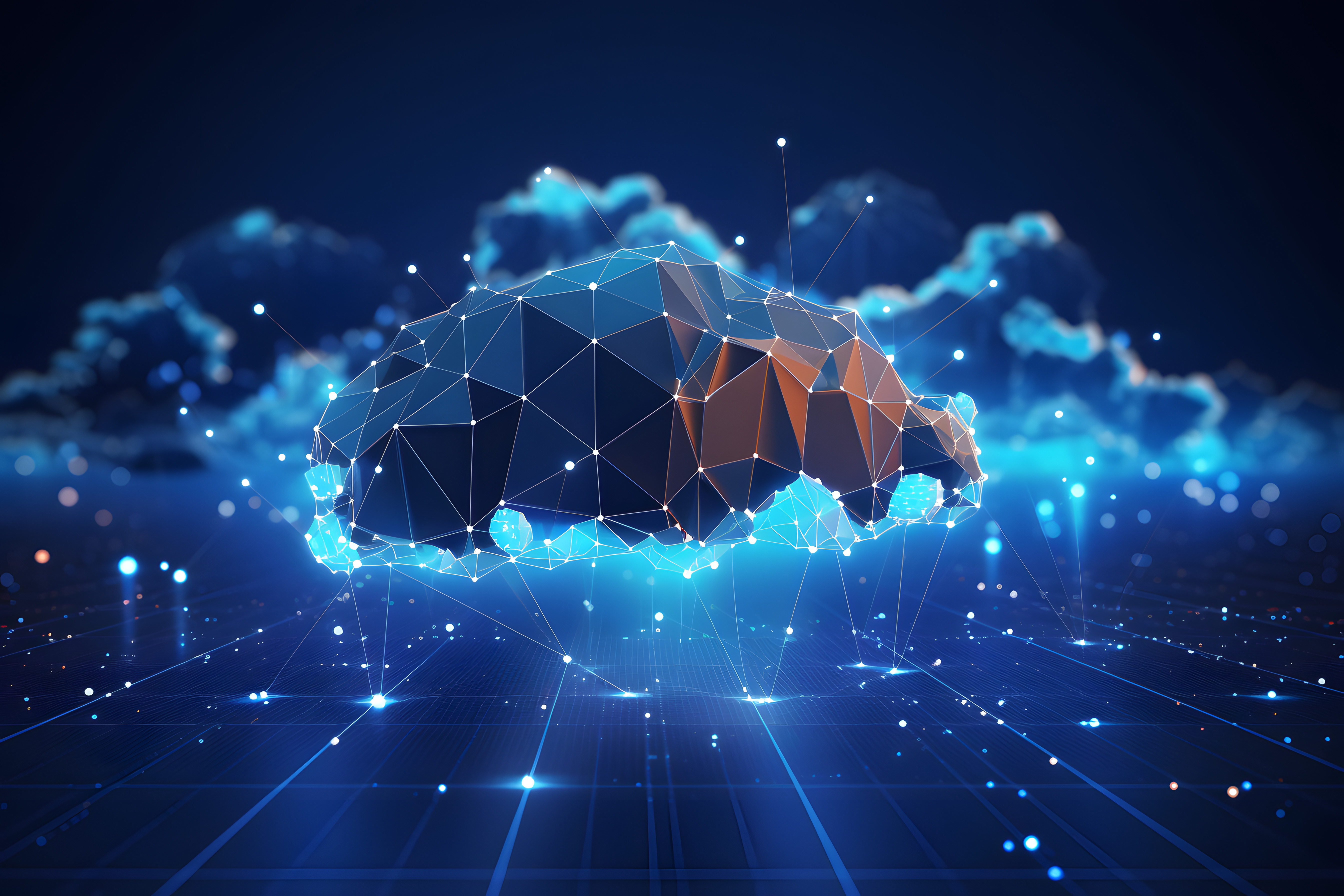
Nervous About Where VMware Is Going? Here Are Some Paths Forward
When chipmaker Broadcom acquired VMware for a cool $61 billion this past November, one of the first questions from many companies was how the acquisition would change VMware’s pricing.
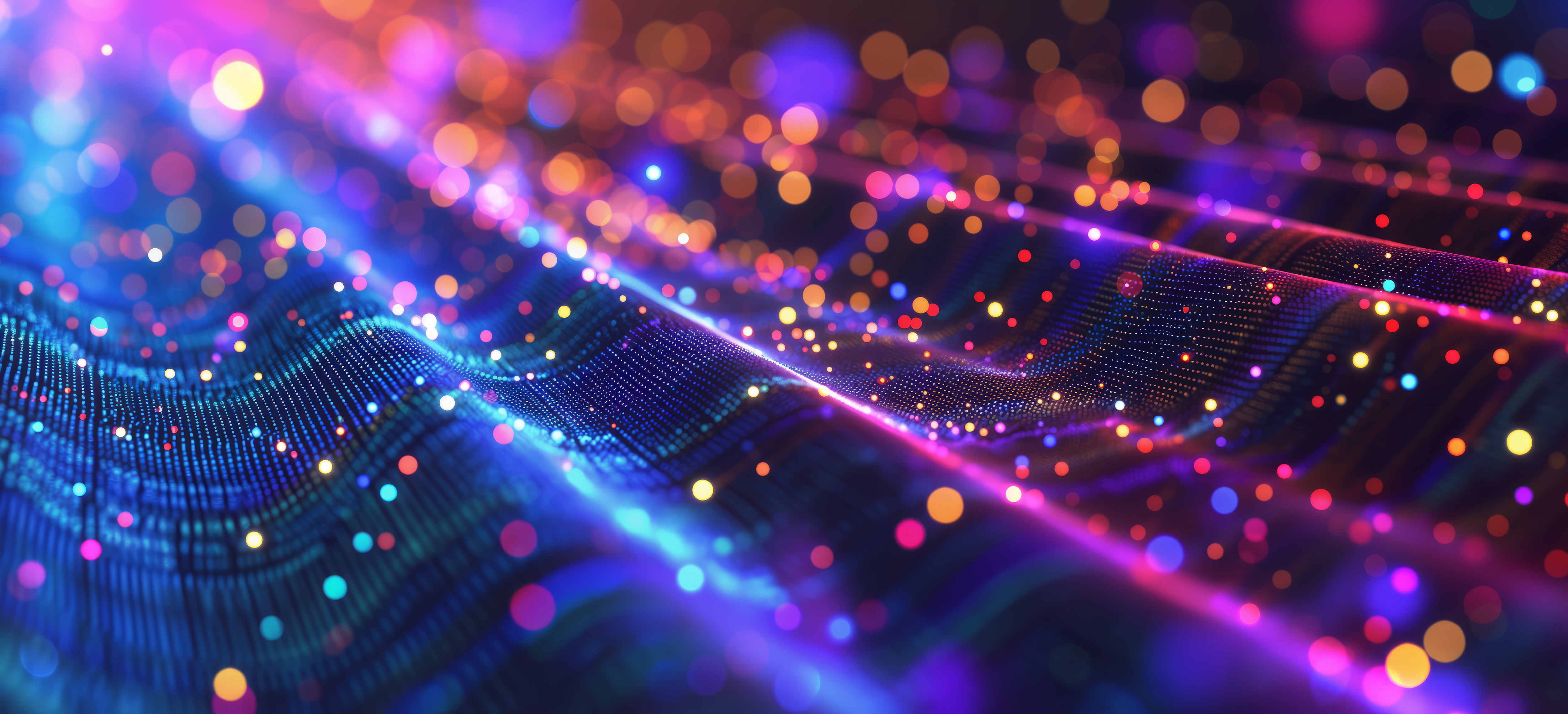
Parallel Processing in Synapse Notebooks
Parallel processing refers to an operational process divided into parts and executed simultaneously across different compute resources to help optimize performance and decrease load times. This is...
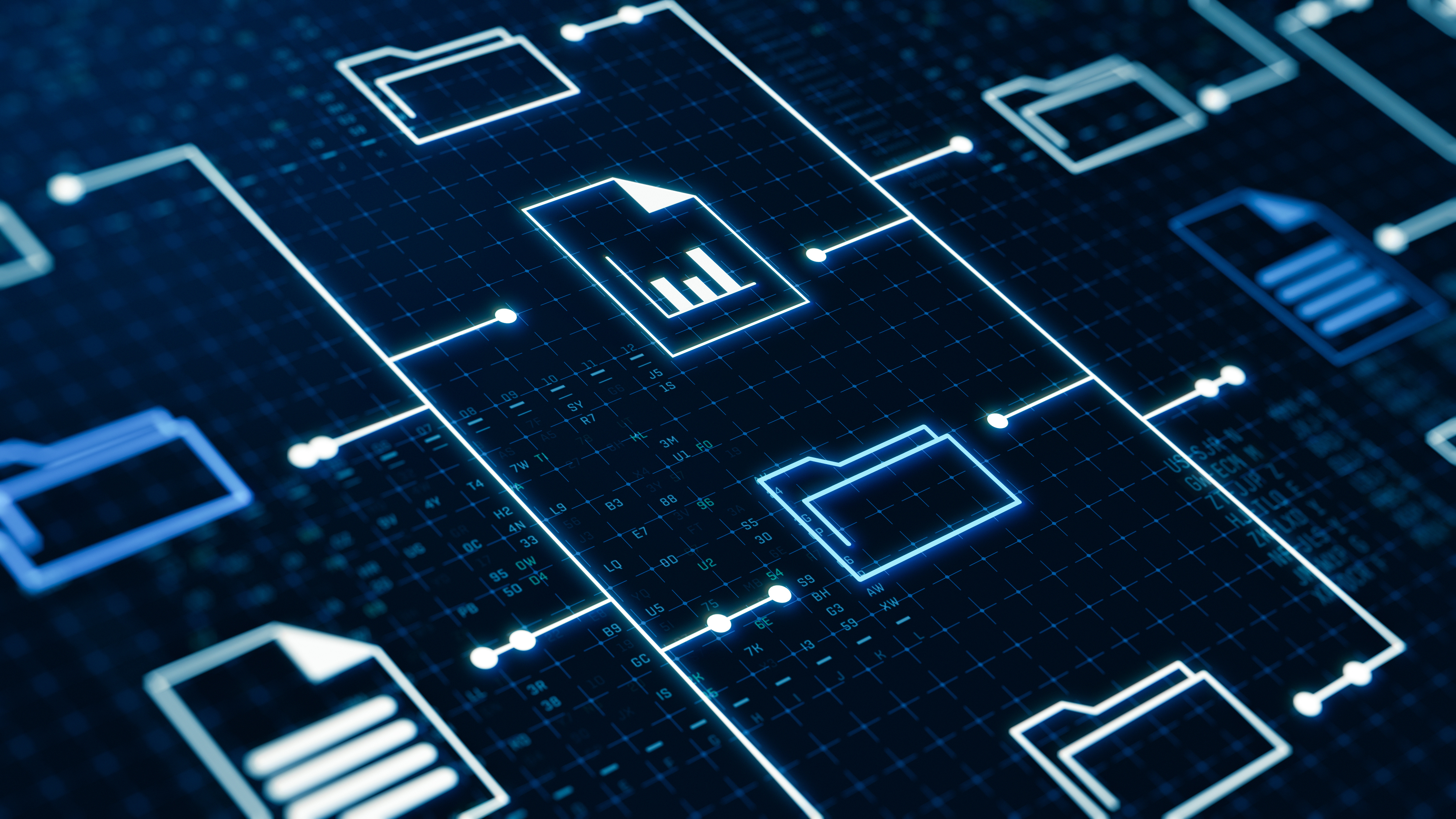
Storage in the Cloud
Public cloud storage promises agility, scalability, and endless capacity. However, for many organizations, the reality falls short. Limited performance, capacity constraints, and skyrocketing costs...
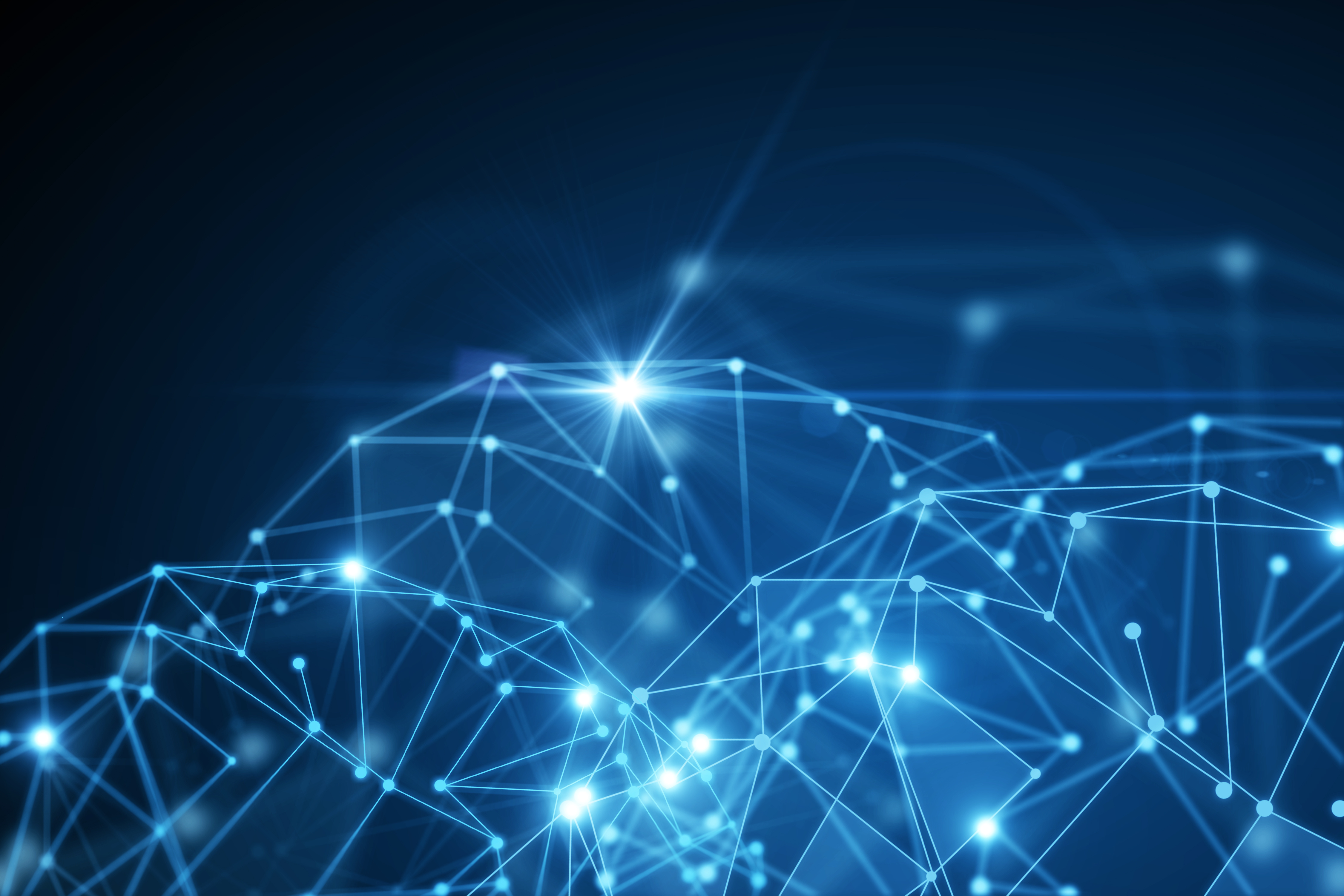
The Crucial Role of Cost Optimization in Digital Transformation and the AWS Well-Architected Framework
As organizations embrace containerization, the significance of efficient cost optimization emerges as a critical component for successful digital transformation. Our upcoming webinar on Container...